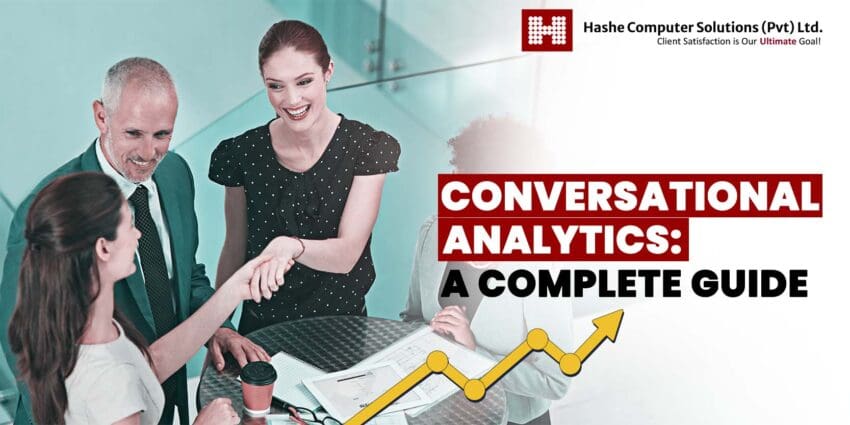
As organizations grow more digital, communications occur through chatbots, social media, emails, and messaging applications. This generates copious amounts of data that could be valuable to the company.
Finding the correct insights about your products, services, and experiences could be challenging while dealing with customers through various channels. That’s where conversational analytics comes in.
By analyzing all of the interactions that take place via the phone, email, live chat, social media, and other channels, conversational analytics enables you to discover real-time consumer sentiment and emotion and make the required adjustments.
This article delves into conversational analytics, discussing how it works, its advantages, potential drawbacks, and how to use it in your company so you can make informed decisions about improving customer experiences and products.
What is Conversational Analysis?
Conversational analytics is the technique of analyzing customer conversations to gain insights into their behavior, preferences, and general sentiment. Its goal is to use this information to personalize future conversations, increasing consumer satisfaction and engagement.
Conversational analytics transcribes and analyzes thousands of voice calls, chat conversations, social media activities, and more using Artificial Intelligence (AI) techniques like Machine Learning, Natural Language Processing (NLP), and different algorithms.
Businesses may identify trends in consumer behavior, assess sentiment, and enhance customer experiences by studying these interactions at scale.
Key Components of Conversational Analytics
- Natural Language Processing (NLP): Natural language processing (NLP) is a subfield of artificial intelligence (AI) that facilitates computer comprehension and interpretation of human language. Conversational analytics depends largely on NLP techniques for extracting meaning and context from text or voice input.
- Sentiment Analysis: It entails identifying the consumer sentiment or tone expressed in human speech. It aids companies in determining client satisfaction levels and possible concerns or challenges.
- Intent Recognition: Intent recognition involves understanding the purpose or objective of a customer’s query or request. It enables organizations to give relevant responses and increase the success of conversational interactions.
- Performance Monitoring: Companies can monitor the performance of their conversational interfaces via conversation analytics software. This entails tracking KPIs such as resolution rates and response times to pinpoint areas for development.
- Customer Journey Analysis: Conversational analytics help examine customer interactions across numerous touchpoints and acquire insights about their whole journey with the company.
- Topic Extraction: Conversational analytics can determine the primary topics or subjects of a conversation. It aids companies in concentrating on the most pertinent problems and seeing patterns or trends in client queries.
- Recommendations and Personalization: Businesses can use conversation analysis to customize responses and recommendations based on consumer behavior and preferences.
How does Conversational Analysis Work?
Businesses can enhance customer experiences and operational efficiency by using conversational analytics to understand consumer demands, optimize interactions, and make data-driven decisions. Here is how it works.
Data Collection
The initial step is to acquire conversational data from numerous touch points throughout the client journey. These could include transcripts from live chats, emails, phone conversations, social media postings, comments, and direct messages.
Understanding the Language
The system uses Natural Language Processing (NLP) to turn speech into text during spoken conversations. NLP assists the system in deciphering the meaning of the user’s words, including their intent and sentiment.
Combining Data Sources
Conversational analytics tools blend traditional analytics data sources such as CRM, call center software, and knowledge bases to provide a comprehensive and holistic perspective of your consumers. The software recognizes interactions with the same client across several devices and applications via datasets and algorithms.
Analyze Data with AI
Machine learning (ML) and artificial intelligence (AI) algorithms then examine the text data. These algorithms are trained to recognize emotions, keywords, and conversation patterns
Obtaining Practical Insights
The system finds insights like customer satisfaction scores (CSAT), prevalent or trending concerns, and customer behaviors by examining conversations and client queries. Additionally, it displays essential call center KPIs and metrics, including initial response times and first contact resolution rates.
Interpreting the Data
Finally, the conversation analytics tool converts this extensive data into understandable reports and graphics. You may quickly identify patterns and trends in all of your customer contacts with the help of these reports.
Differences Between Conversational Analytics and Traditional Analytics
The typical contact center uses voice as the sole or primary service channel. Their primary contact center KPI data source is sales conversations, including average call times, wait durations, and first call resolution. Businesses traditionally evaluate operational effectiveness and customer service experience using text analytics and statistical analysis. Since the data is obtained in pieces and processed separately, the traditional technique for analyzing conversations limits the quality and scope of insight.
With the development of artificial intelligence (AI) and other new technologies, businesses may now utilize software designed specifically to examine every communication channel in contact centers. Gaining far more thorough insights is now feasible because of the tools and AI that arrange, analyze, and display the data from chatbots, emails, text messages, and phone conversations.
Conversational analytics is more in-depth and timely than traditional analytics. Traditional analytics use text analytics approaches to extract insights from past sales discussions, providing a high-level and comprehensive perspective of the call center. It might help you comprehend long-term patterns and contact center dynamics. However, the insights may soon become outdated because the data is collected gradually from past data.
Conversational analytics provides comprehensive business insights by leveraging AI to record and analyze customer-agent interactions. Managers can get rapid oversight of agent performance, call volume trends, customer happiness, and other aspects of the business with the use of real-time data analysis.
Conversational analytics insights can be beneficial in helping agents make better decisions during live encounters, developing a deeper understanding of the client, and cutting expenses by using data-driven problem-solving techniques. Traditional contact center analytics are unable to provide insights of this caliber.
Aspect | Conversational Analytics | Traditional Analytics |
---|---|---|
Scope | All customer-agent interactions and CRM data | Phone conversations and customer profiles |
Data Source | Recent conversations across calls, chat, text message, emails (e.g., customer-agent interactions) | Historical records (e.g., sales, customer profiles) |
Real-Time | Yes, allows instant intervention and improvements | Primarily retrospective; does not offer real-time monitoring or intervention. |
Metrics | Customer satisfaction (CSAT) and average handle time (AHT) | Revenue, customer churn, conversion rates and overall business efficiency |
Decision Making | Governs decisions related to customer service quality and agent training | Allows marketing, sales, and customer service to make data-driven decisions. |
Technology | Relies on AI, natural language processing (NLP), and machine learning (ML) | Typically relies on structured data analysis tools, reporting software and historical databases |
Use Cases | Used to analyze customer service quality, customer sentiment and agent performance | Used to assess website performance, e-commerce sales and marketing campaign impact |
Difference Between Speech Analytics and Conversational Analytics
Despite having somewhat different focuses, both conversational analytics and speech analytics gather insights from consumer conversations.
Speech analytics evaluates the acoustic characteristics of speech, such as tone, pitch, and speed, as well as the words stated during customer care conversations. Its primary function is to gauge agent performance and call quality.
Conversational analytics extends beyond voice to chats, texts, and other modes of communication. In addition to the acoustic characteristics, the analysis considers the interaction’s context, the sentiment, and the intention of the talks.
Traditionally, speech analytics methods were simple, parsing text for keywords. Conversational analytics offers a more comprehensive perspective of interactions with the advent of AI, helping the contact center to meet its objectives.
What are the Benefits of Conversational Analytics?
Conversational analytics’ goal is to fully understand your customers—it’s not just about listening to them. Analyzing client interactions can provide valuable insights and customer feedback, allowing you to make business decisions that continually deliver improved customer experiences.
Here are the most significant benefits of conversational analytics.
Enhances Sales and Conversions
Imagine being able to identify the points in a sales conversation where prospects are most likely to become customers. Sales teams can increase their ability to complete transactions by using conversational analytics to monitor sales conversations and uncover buying signals and client objections. By being aware of these obstacles, you can improve how you handle sales objections and increase conversion rates.
Boosts Customer Experience
Analyzing customer conversations might help you spot potential weak points in their experience. You may identify client pain areas and take proactive measures to alleviate them by studying sentiment across various platforms. It could include expanding your self-service alternatives and optimizing customer support operations, such as improving average handling time (AHT).
Improves Agent Performance
Your customer service representatives work on the front lines, connecting with customers daily. Analyzing call recordings and chat transcripts helps you identify areas where agents should improve, such as resolving customer escalations or adhering to particular standard operating procedures.
Afterward, you can use this information to track agent performance indicators and give your agents customized coaching and training. It enables you to share the best practices of high performers with the rest of the team and observe what they are doing right to succeed.
Helps Gain Competitive Edge
Business is a highly competitive environment. You need any advantage you can get. You will gain a deeper understanding of your customers with the help of conversational analytics. You will have a clear picture of their wants and preferences. Then, you may outsmart your rivals by customizing your marketing, goods, and services to meet their needs.
Conversational Analytics Use Cases
Customer Support
Conversational analytics can help examine how customers engage with chatbots, virtual assistants, and call center personnel. Businesses can use it to track agent performance, find frequent client problems, and speed up response times for better customer service.
Voice of the Customer (VoC) Analysis
Businesses can analyze client feedback from various conversational channels, such as phone calls to a contact center or chatbot interactions, to learn more about their customers’ preferences, problems, and general attitudes toward their products and services.
Sales and Marketing Optimization
Conversational analytics can help you comprehend client inquiries during sales interactions. Businesses can use it to find possible cross- or up-sell possibilities and to tailor marketing messages to the preferences of their target audience.
Personalization and Customer Journey Mapping
Analyzing client conversations can assist in developing personalized experiences based on individual interests and behavior. It also helps with customer journey mapping, which raises engagement and retention.
Risk Management and Fraud Detection
Conversational analytics can improve security measures in financial institutions by assisting in the detection of suspicious activity or fraudulent behavior during customer interactions.
Compliance Monitoring
Conversational analytics solutions can help monitor regulatory and internal policy compliance during customer interactions, ensuring adherence to industry standards.
How to Implement Conversation Analytics
Although conversational analytics seems like a terrific tool with endless potential for your company, its actual impact will largely depend on how you use it. Here’s a step-by-step guide for successfully incorporating conversational analytics into your contact center operations.
1. Define Scopes and Objectives
Outline the goals for employing conversational analytics. Establish your goals, such as raising consumer insights, maximizing agent performance, or boosting customer service. Define the extent and the channels (phone, chat, email) that will be covered.
2. Select the Right Analytics Platform
Choose a conversational analytics platform whose scope and aims match your customer service goals. Ensure that it has all the required functionality, such as the capacity to analyze data from several channels and real-time monitoring.
3. Data Collection and Preprocessing
Collect a sample of consumer interaction data from each channel. Ensure that the data is well-structured and spans a reasonable period. Clean and pre-process collected data to remove noise and extraneous information while maintaining uniform formatting.
4. Speech-to-Text Conversion
If voice call analysis is your goal, use speech-to-text technology to translate audio conversations into text. The Automatic Speech Recognition (ASR) technology makes it feasible. With algorithms trained on copious amounts of audio data, ASR is highly proficient in identifying speech patterns and translating spoken words into text. It plays a critical role in making voice data accessible and searchable.
Conversely, use Text-to-Speech (TTS) as a vocal communicator. TTS brings the written text to life, making it an essential tool for systems such as voice bots that need to engage consumers verbally, assuring seamless communication and improving the customer experience.
5. Sentiment Analysis
Use sentiment analysis to assess customer sentiment during conversations. Determine whether the sentiment is positive, negative, or neutral.
To accomplish this, use a sophisticated technique that combines machine learning, statistics, and NLP to uncover both expressed and unspoken customer sentiments during encounters. Various sorts of algorithms drive sentiment analysis models.
- Rule-Based Approach: It describes polarized words in the text as positive, negative, or neutral. It counts polarized words and detects attitudes via NLP techniques to create personalized rules.
- Automatic or ML-Based Sentiment Analysis: Machine learning algorithms are trained on datasets that include both positive and negative words. After training, these algorithms can identify the tone and sentiment of the text automatically.
- Hybrid Sentiment Analysis: It blends sentiment analysis techniques from both automatic and manual sources. This method is well known for yielding incredibly precise outcomes.
6. Real Time Monitoring
Use real-time monitoring to spot problems as they occur. It enables agents to intervene immediately. These insights are not limited to a single format or platform; you can easily share them throughout your organization in various formats and on any device.
7. Agent Performance Analysis
Leverage agent performance metrics, such as response time, script adherence, AHT, and issue resolution, to assess the agent’s performance during the conversations. Dashboards and reports may visually show these data elements. You may see a comprehensive overview of your agents’ daily, monthly, and quarterly performance.
8. Customer Insights
Understand customer preferences and issues. Identify recurring issues and possibilities for proactive help by analyzing data points displayed on dashboards and reports.
9. Compliance and Data Security
Ensure the implementation meets data privacy and security laws while guaranteeing consumer data integrity.
Key Metrics and KPIs for Conversational Analytics
Sentiment Score
Sentiment score is a crucial conversational analytics statistic that signifies the emotional tone of consumer interactions. This metric uses the words and context to determine if the sentiment is positive, negative, or neutral.
Keeping an eye on sentiment scores can enable you to gauge client happiness and identify problems before they get out of hand.
Customer Effort Score (CES)
The Customer Effort Score (CES) evaluates the ease of contact from the customer’s perspective. A low effort score implies clients can get their problems resolved easily, signifying higher satisfaction.
Tracking CES would help you discover pain points in the customer journey and focus on improvement.
Net Promoter Score (NPS)
The Net Promoter Score (NPS) assesses client loyalty by asking them how likely they are to suggest your products or services. NPS is a reliable predictor of future business growth and a good measure of customer happiness.
By using conversational analytics to track NPS, you can determine the efficacy of your client retention campaigns.
First Response Time and Resolution Time
The first response time is the time lag between a customer’s inquiry and the business’s response. The time it takes to fix a customer’s problem after initial contact is known as the resolution time.
When evaluating customer support teams, these indicators are essential. Improving these times will typically result in happier customers.
Challenges for Conversational Analytics
Although conversational analytics is a powerful and promising tool, its proper deployment and efficient use require addressing some issues. Some of the primary challenges include:
Uncertainty and Variability of Natural Language
Natural language is fundamentally vague and varies widely among individuals. Slang, colloquial language, and irregular grammar might be present in conversations, which makes it more difficult for NLP algorithms to comprehend sentiment and intent.
Context Sensitivity
Comprehending the context is essential for providing insightful answers during discussions. However, acquiring and keeping context throughout a conversation can be difficult, particularly in multi-turn interactions.
Data Quality and Noise
Conversational data may be noisy and contain typos, misspellings, or inaccurate data. Poor quality data can skew findings and impact the accuracy of NLP models.
Training Data Bias
NLP models could be biased because they are trained on historical data from previous interactions. Biased training data might produce biased answers, either harming or reinforcing stereotypes.
Scalability and Performance
Managing a large number of real-time discussions necessitates scalable, high-performance infrastructure. NLP algorithms’ processing speed can make it difficult to maintain responsive conversational interfaces.
Privacy and Data Protection
Conversational analytics entails evaluating sensitive consumer interactions. Data privacy and compliance with data protection requirements are critical, but finding a balance between offering personalized responses and protecting client information can be challenging.
Addressing these problems will necessitate ongoing research, NLP and AI technology innovations, and a careful approach to data collection, model training, and system design. Organizations that successfully navigate these obstacles will reap the numerous advantages of conversational analytics solutions.
Wrapping Up!
You may extract more value from your consumer data by using conversational analytics. Based on real data and KPIs, you may obtain a more accurate picture of agent performance with improved attribution and customized insights.
Are you looking for the best IT providers for your IT projects? Look no further than Hashe! Hashe Computer Solutions is a leading IT solutions provider that offers world-class software, mobile application, web development, and digital marketing services. Contact us for the best web design solutions!
Keep following us for more tech news!
Check out our Social Media Pages:
- Facebook: https://www.facebook.com/hasheCS/
- Instagram: @hashe_official
- LinkedIn: https://pk.linkedin.com/company/hashe-computer-solutions
- Twitter: https://twitter.com/hashe
- Youtube: https://www.youtube.com/channel/UCay7eQtwoQ3ehoIesAxHDPw
- Pinterest: https://www.pinterest.com/hashecs/
- Threads: https://www.threads.net/@hashe_official
- Behance: https://www.behance.net/hashecs
Was this helpful?
Last Modified: September 23, 2024 at 7:54 am
28 views